Machine Learning in Healthcare: Applications and Challenges
- Date August 30, 2023
Machine learning (ML), a subset of artificial intelligence (AI), has contributed to several beneficial advances in the healthcare industry. The market for AI in healthcare will grow from 14.6 billion USD in 2023 to 102.7 billion USD in 2028 due to AI’s incredible capacity to evaluate and interpret complex medical data.
To produce predictions or judgments, algorithms and models that can learn from data without explicit programming must be created. Many tasks in the healthcare sector can be automated with the help of machine learning (ML), including diagnosing diseases, developing new drugs, and many others.
By employing large datasets and powerful algorithms, machine learning can offer insightful information and improve decision-making in healthcare settings.
How Can Machine Learning Help the Healthcare Industry?
Machine learning is essential for enhancing patient care, healthcare outcomes, and the overall effectiveness of the healthcare system. The following are its benefits for healthcare:
- Enhanced accuracy
ML algorithms can evaluate enormous volumes of medical data and spot significant trends that may not be obvious to human observers. People are, therefore, better able to foresee and recognize events.
Also, the algorithms have proven the capability of correctly identifying anomalies in medical images, hence lowering the risk of misdiagnosis in radiology.
- Drug Discovery & Development
The process of creating new medications is labour-intensive and complex. Machine learning can dramatically speed up this process by examining massive databases of chemical compounds, forecasting their interactions with biological targets, and identifying prospective drug candidates.
Doing so makes it possible for researchers to first focus on evaluating the most promising substances.
- Personalized Treatment Recommendation
To find patterns and associations, machine learning can evaluate enormous datasets of patient characteristics, medical histories, genetic data, and treatment outcomes. Through ML, it is possible to create unique treatment programs for each patient.
Applications of Machine Learning in Healthcare
Disease diagnosis and prognosis
You can use automated analysis of medical images such as X-rays, MRIs and CT scans to diagnose and predict diseases. Machine learning algorithms have demonstrated excellent capabilities in this regard. With these algorithms, photo patterns and anomalies can be used to diagnose diseases.
Radiologists, for instance, can use machine learning algorithms to spot early symptoms of conditions like cancer, neurological issues, and cardiovascular disorders.
DeepMind Health, a Google ML product, has been trained to dissect retinal reviews and look for signs of diabetic retinopathy. It enables medical professionals to pinpoint the initial stages of retinal degeneration, allowing for early intervention and forestallment of diabetic vision loss.
To find early signs of complaint, machine literacy algorithms can search through various data sources, similar as medical records, inheritable information, and life data.
By fitting these bitsy suggestions and taking prompt action, healthcare providers can ameliorate patient prognostic and treatment effectiveness.
Delivering personalized care
For predicting remedies, machine literacy looks at vast databases of patient characteristics, available curatives, and remedial issues to induce personalized treatment recommendations. Medical experts can choose suitable treatments for certain patient cases based on the probable impacts of various treatment options.
For example, Using data from the brain’s connectome, or the “wiring” between neurons, and machine learning, an international team of researchers led by EPFL has created a method to evaluate and forecast the outcomes of stroke sufferers. By taking this action, doctors can predict whether a case will recover entirely and design customized surgical plans for them.
Drug discovery and development
- Compound virtual screening
Machine learning algorithms can examine massive chemical databases and forecast a compound’s probability of binding to a particular target. Identifying new medication candidates can be made much faster and cheaper with this virtual screening procedure than with conventional drug discovery techniques.
For example, Atomwise, a company specializing in machine learning for medicine discovery, successfully linked implicit medicine campaigners for Ebola and multiple sclerosis. Their algorithms screened millions of composites and prognosticated their list affinity to specific proteins associated with these conditions, helping experimenters prioritize the most promising campaigners for further discourse.
- Optimization of medicine phrasings
Machine literacy can help optimize medicine phrasings by assaying data on medicine parcels, delivery systems, and patient responses. By relating patterns and correlations in these datasets, machine literacy algorithms can guide the expression process to enhance medicine efficacy, reduce side goods, and lessen patient compliance.
Patient monitoring and prophetic analytics
- Remote case monitoring
Machine literacy algorithms can dissect real-time data from wearable bias, like heart rate observers, glucose measures, and exertion trackers, to recover a patient’s health. These algorithms can describe patterns and anomalies in the data, enabling early intervention and substantiated care.
- Real-time health monitoring and caution
Machine literacy algorithms can dissect patient data in real-time to give immediate cautions and warnings. For illustration, machine learning models can reuse multiple physiological parameters in ferocious care units, like heart rate, blood pressure, and oxygen achromatism, to predict the liability of critical events like sepsis or cardiac arrest.
Healthcare operations and administration
Challenges of Machine Learning in Healthcare
Enforcing machine learning in healthcare has several challenges that must be addressed for successful relinquishment and integration into healthcare systems.
Data Quality and Availability
Machine learning models process significant, different, high-quality datasets to learn effectively. Still, healthcare data is frequently disintegrated across other systems, needs more standardization, and may have missing or deficient information. Also, it ensures data sequestration and security while making it accessible for analysis poses a significant challenge.
Interpretability and Explainability of Models
Machine learning models can be considered” black boxes” because their decision-making processes aren’t fluently accessible by humans. In healthcare, interpretability and explainability are pivotal to gaining trust and acceptance from healthcare professionals.
Developing ways that give perceptivity to the factors contributing to a model’s predictions or decisions is crucial.
Regulatory and Ethical Considerations
The healthcare sector is subject to strict regulations, similar to the Health Insurance Portability and Accountability Act( HIPAA) in the United States. Enforcing ML in healthcare requires compliance with these regulations to ensure patient consent and privacy.
The ethical issues of algorithmic bias, algorithmic fairness, and implicit patient data exploitation must also be addressed.
Integrating with existing systems like electronic health records( EHR) or clinical information systems might be difficult because of interoperability problems, different data formats, and a need for IT structure. The successful usage and implementation of machine learning in healthcare depend on easy integration.
Trust and Human Factors
For machine learning to succeed, healthcare professionals and patients must come on one page. It’s pivotal to establish transparency, responsibility, and clear communication regarding the capabilities and restrictions of machine knowledge algorithms.
Enforcing the ML tools in hospitals successfully depends on training the healthcare labour force with 24*7 technical support.
Conclusion
Machine learning has the implicit ability to advance healthcare services through predicting opinions, personalized treatments, expediting the discovery of new drugs, perfecting patient monitoring, and streamlining executive processes.
To ultimately realize the advantages of machine learning in healthcare, it’s still necessary to address issues with data sharing, interpretability, regulatory compliance, integration, and human aspects.
Collaboration between specialists in the field of healthcare and technology is essential to curb these issues and overcome the challenges of ML in healthcare.
Previous post
Data Structure Design: Principles and Best Practices for Creating Efficient and Scalable Data Structures
Next post
The Role of Explainable Artificial Intelligence (XAI) in Building Trustworthy AI Systems
You may also like
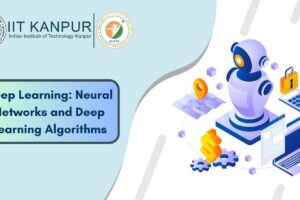
Deep Learning: Neural Networks and Deep Learning Algorithms
